Cooperatives Should Embrace the Opportunities of AI

Establishing a shared definition for AI has been challenging in recent years. As co-authors of an analytic report on AI research, we identified keywords from research papers, hoping to build a definition, but agreement among experts remained elusive. Now, with ChatGPT and other large language models (LLMs) make AI more tangible for everyone, transcending the common association with robots and science fiction, even enabling understanding for children. Remarkably, ChatGPT onboarded 1 million users in just 5 days, igniting enthusiasm to explore its capabilities. Within 4 months, Microsoft and Google integrated ChatGPT into their app suites, with competitors following suit. Widespread adoption showcases their potential. Yet, we must address copyright concerns, hallucinations, and accuracy, as they demand attention. Mozilla’s venture approach to “building trustworthy and open-source AI” is a promising step. The emergence of the platform economy has given rise to cooperative alternatives that offer participatory, fair, and responsible alternatives. Ride-hailing cooperatives, for instance, have recently gained prominence as a prime example of platform cooperatives. Although we observe the emergence of data cooperatives as a cooperative format, there appears to be relatively less emphasis on AI within the cooperative movement.
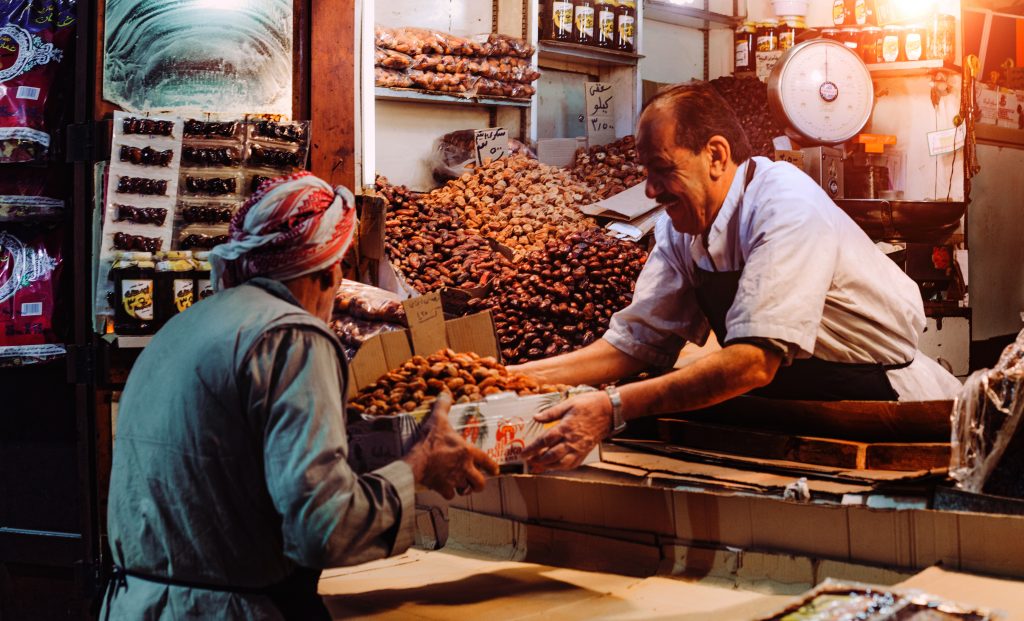
Throughout the period of industrialization, cooperatives were formed to address various needs, including access to quality food, efficient processing of agricultural products, marketing, and finance. The underlying goal was to strike a new balance between consumer-driven goods that were affordable and produced in high volume while maintaining control over working conditions and ensuring a fair distribution of the value created. Indeed, the concerns and claims we face with AI are reminiscent of the threats and issues encountered during industrialization. With AI, we encounter risks such as the loss of control over our data, the proliferation of centralized and biased models, the exploitation of data workers, and unpredictable societal impacts.
There are similarities but the impact on the everyday lives of people is different than it was in industrialization – yet, other than fundamental needs and rights such as nutrition and health. Users today tend to ignore the data and privacy risks until they are directly affected, e.g., in credit scores, job selections, etc. This “privacy paradox,” as it is often referred to, reflects the phenomenon where online users express concern about privacy but exhibit behaviors that contradict those concerns, highlighting the abstract nature of data, AI, and privacy challenges, as usability and convenience often take precedence over less visible risks and downsides, implying that society may require additional time to comprehend the impacts and develop a better understanding of AI’s capabilities and implications.
While governments are indeed developing new regulations, such as the EU’s Data and AI Acts, which involve classifying AI into risk categories and implementing civic liability approaches for new technologies, particularly AI, the question remains whether these measures are sufficient. Let’s consider that AI encounters challenges of similar magnitude as those faced a century ago. That is why, in addition to regulation, cooperatives might be a suitable model. An AI cooperative would be built upon the fundamental components of AI: curated data, code, and use case adaptation. In its simplest form, AI involves the application of algorithms or code to carefully curated datasets, resulting in trained models or supervised approaches. These models are then utilized to address challenges such as prediction, classification, and optimization, by applying them to new data and contexts.
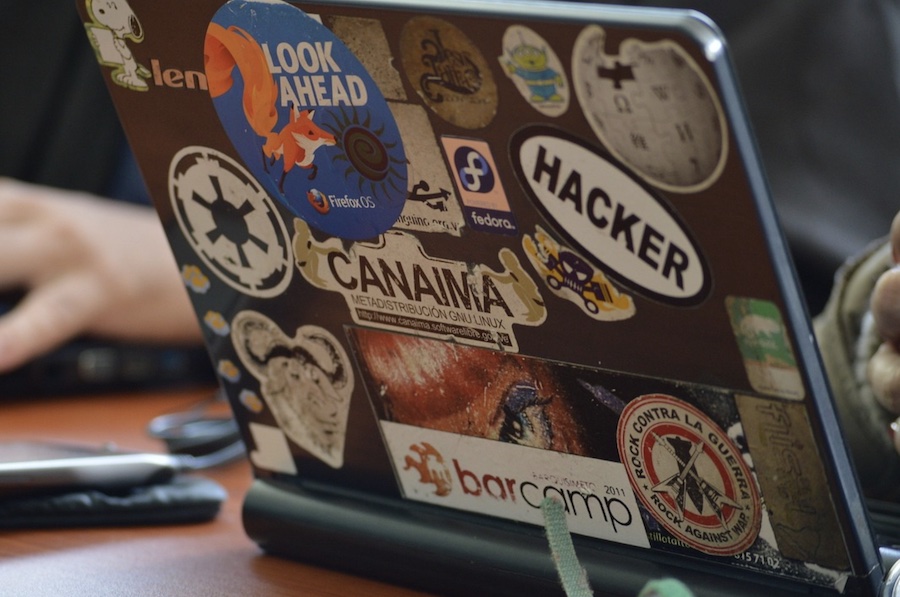
AI cooperatives can be viewed as a combination of data cooperatives and existing cooperatives, where data is shared and code is applied to address specific challenges. However, since much AI code is already available open source and in common use, AI cooperatives may not necessarily need to operate as stand-alone entities. Instead, the focus could be on co-owning the trained models, which data cooperatives could potentially achieve by deriving them from their co-owned data. With the presence of open-source communities, established networks of NGOs and advocacy groups, engaged governments, and the emergence of DAOs in Web3, there are already several alternatives for balancing the impact of AI. This suggests that owning AI cooperatives may not be a necessary requirement, as there are existing avenues to promote responsible AI practices and collaborative efforts.
In practical terms, let’s consider the GPT implementations in Microsoft as an example. Microsoft combines its applications, language models, and the Microsoft Graph to provide enhanced functionality and contextual use cases. While this integration brings productivity benefits, it raises important concerns. One such concern is data ownership, as users’ personal data becomes absorbed and owned within the Microsoft Graph. Additionally, users may have to pay for enhanced features without receiving direct compensation for their data contributions. Furthermore, there is the issue of user data being potentially used to train other models and AIs in which the user has no participation.
Addressing data sovereignty and the value creation of users is crucial. Data cooperatives or DataUnions empower users to control their data and actively participate in its value generation. This evolution could lead to digital asset cooperatives, where code and resulting models are collectively owned and managed. This approach enables users to have a stake in the value created by AI technologies while retaining control over their personal data.
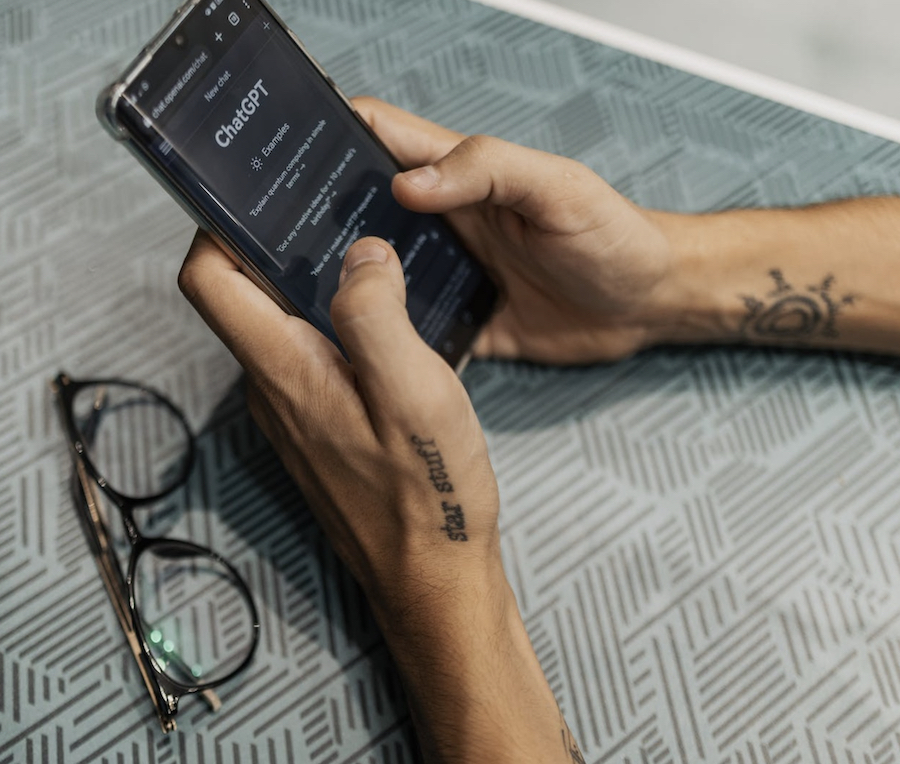
Using the prompt (Feb 2023) I asked: “write a thought provoking piece for discussion on the role, opportunities, and challenges of cooperatives for AI to help democratise AI and allow more people to participate in AI”). ChatGPT responded that cooperatives have the potential to democratize AI and facilitate broader participation in its development and deployment. They can enable collaboration, resource pooling, and collective decision-making. Key opportunities for AI cooperatives include aligning AI technologies with community values, providing access to resources and expertise, fostering fairness and equitable distribution of benefits, and promoting democratic and transparent models of AI. However, there are challenges to address, such as securing funding, acquiring technical expertise, competing with established AI entities, and managing governance and decision-making processes. The output provided by ChatGPT aligns well with earlier considerations.
What follows is a set of reflections and tentative recommendations.
Integrating AI into existing cooperatives
Embedding AI and data capabilities within existing cooperatives, such as platform coops or international coordinating organizations, enables the integration of AI into cooperative frameworks, while data cooperatives can extend their focus to include AI. Additionally, cooperatives can establish Coop-facilitated DataUnions or DataDAOs, fostering collaboration and leveraging the expertise of established cooperative entities.
Cooperatives foster fair participation in sustainable, value-driven economic frameworks
Cooperatives can embrace cooperative economies aligned with democratic governance, value sharing, and mutual benefit, such as Open Source, Web3 DAOs, data protocols, data blockchains, and data marketplaces. By actively participating in these ecosystems, cooperatives can connect their values, represent local and domain contexts, and explore new income sources. This involvement enables cooperatives to contribute to the development of fair and sustainable cooperative models within these emerging economic frameworks.
Cooperatives leverage AI and data opportunities.
Cooperatives leverage AI and data opportunities as stewards, governing data and participating in model ecosystems for development and engagement.
Cooperatives benefit from utilizing data and AI for decision-making and operations.
It is essential for cooperatives to explore the power of data and AI for their own decision-making and operations, beginning with the utilization of their own member data. This empowers cooperatives to harness the benefits of data-driven insights and AI technologies in their internal processes, enabling them to make informed decisions and enhance their operational efficiency.
Cooperative economies and alternative models provide pathways for leveraging the benefits of data and AI. They can actively contribute to AI economies, benefiting from crowd-powered software development and expertise. Lastly, they can take on the role of data stewards, participate in model governance, and integrate data and AI into their own operations.